PLANNING TO SHARE QUALITATIVE DATA
Sharing research data can be simple when a data sharing plan is established at the earliest stages of study planning. Even if a research project did not plan for data sharing at the onset of the study, data sharing is still possible and may be required by the funding agency or sponsor.
This planning guide provides guidance and key considerations for research project planning, data collection and management, and depositing qualitative research data in a data repository. This guide focuses on the US context; however, we have added some resources at the end of the guide that may serve qualitative researchers in other nations.
1. Clarify What “Data Sharing” Means for Your Project.
Data sharing requirements and plans will vary depending on the context of a research project. Funding agencies, journals, and data repositories will set their own policies and procedures for data sharing. Always review the data sharing policies of a project’s current or projected funding agency when determining what data sharing will look like for the project.
Files to Share. Ordinarily, sharing audio or video files with secondary users is not recommended unless required by the project’s funding agency or desired by participants (e.g., in an oral history project). Unlike written transcripts, audio and video files from interviews or focus groups are often impossible to fully de-identify. However, even when audio or video files are not shared with secondary users, it may be prudent to retain these files in study records to ensure data integrity. Funding agencies, research institutions, and professional associations have their own standards for how long study data must be retained after data collection concludes.
Below we provide a checklist of data files and supporting documentation generally included in a data deposit.
- Study Description. Study descriptions are valuable resources to data users. They include general information, such as study title, funding, investigators, and summary of the study purpose. A study description should also include more detailed information about the study design, methodology, and research practices. Include information about how the data was transcribed and de-identified. You may want to attach the study protocol to provide more information about data collection and analysis.
- Project Documents. Include blank copies of the IRB-approved consent form, recruitment materials, and the IRB protocol.
- De-Identified Data Files. This includes the de-identified transcripts from the qualitative data collection and any data accompanying the qualitative data, such as de-identified survey data. Ordinarily, this will not include identifiable data, such as raw transcript files or audio or video files from data collection.
- Data Collection Instruments. This includes blank copies of any instruments used to collect participant data, such as interview guides and surveys.
- Data Analysis Instruments. This includes copies of codebooks used to analyze the qualitative data, as well as variable codebooks for any accompanying quantitative data. Include definitions for each code or variable used for analysis, and indicate any variables used to identify data or link different data sources together, such as a participant ID. You may or may not want to share your code applications; check with your repository or funding agency to clarify expectations, and follow the terms that were agreed upon in any funding plans or data sharing agreements. At a minimum, the study team must retain code applications in study records.
- Citations for the Dataset. Include a bibliography of any existing publications for the dataset.
Secondary User Access. Data repositories may be able to provide open-access or restricted-access data sharing options.
- Open-Access data sharing means that all data and supporting documentation are publicly available. Secondary users are not required to provide any qualifications to access the dataset, but they may agree to generic terms of use required by the repository. Open-access data sharing is not appropriate for sensitive or potentially identifiable data. There are no accepted standards to determine when qualitative data are adequately de-identified. Because of this, open-access data sharing should only be considered if the data are not sensitive and re-identification is unlikely.
- Restricted-Access data sharing means that secondary users are required to meet certain qualifications before they are approved to access data. These may include having IRB approval, providing a research plan, and holding a terminal degree in a relevant field. Repositories may be able to offer more than one type of restricted access for highly sensitive data, such as requiring secondary users to access the data on the repository’s server rather than making the data available for download. Because qualitative data is often sensitive and difficult to fully de-identify, restricted-access is often the most appropriate way to share qualitative data.
- Note that restricted-access data sharing may require additional data sharing agreements to be executed before completing a data deposit. The Execute Data Sharing Agreements section discusses these agreements in more detail.
Dr. Cruz is a medical anthropologist who studies the childbirth practices of women in a remote village in Northern Ireland. She lived in the village from 2016-2018 and observed or assisted in more than 40 births. Now, she is preparing to publish her findings, focusing on the high rate of home births due to mistrust of the local healthcare facilities. One of the journals she would like to publish in requires data sharing. The research participants originally consented to sharing their de-identified data. Still, Dr. Cruz is unsure if she should share this data given the small community, the possibility of re-identification, and the political volatility of Northern Ireland.
Options for Resolution:
Dr. Cruz could consider depositing her data under a restricted use agreement. With a restricted use agreement, secondary users agree to access the data with specific limitations to ensure that the data will be used appropriately. For example, a restricted use agreement might require all secondary users to be principal investigators at an accredited institution. The agreement may also require an IRB protocol and would routinely prohibit any attempt to re-identify or recontact participants. By restricting access to the data, Dr. Cruz can satisfy data sharing requirements while still ensuring data protection.
Dr. Okedina conducted interviews with men who have sex with men in Nigeria. During interviews, the participants discuss having sex with other men, where they meet, and whether their partners (often wives) know about their “other lives.” Homosexuality is criminalized in Nigeria, so Dr. Okedina worries that sharing this information (even if de-identified) could result in harm to the participants if details that are left in the transcripts could identify a participant when considered collectively. |
Options for Resolution:
Dr. Okedina was specifically asking participants about same-sex relationships as part of his data analysis, and this information could result in participants being imprisoned if they were identified. While Dr. Okedina may be able to de-identify the interview transcripts to meet regulatory standards, ensuring the complete anonymization of qualitative data is difficult. Remember that de-identification is only one data protection tool. When sensitive data must be shared, using multiple data protection options, such as sharing under restricted access, is best. Whether and how to share highly sensitive data is a decision that may require input from the participant community, and these decisions need to be transparent to participants during the informed consent process.
In this case, Dr. Okedina has several options to consider: he can discuss with his funding agency whether it can grant a waiver of its data-sharing requirements. If Dr. Okedina moves forward with sharing this dataset, the data may need to be more heavily redacted, removing more than the minimum required information to meet regulatory standards. Dr. Okedina can additionally share his dataset in a repository under the highest level of restricted access.
2. Include a Data Management & Sharing Plan in the Funding Proposal
The specific requirements of data sharing plans will depend on the funding agency. Learn about the data sharing policies of the funding agency and check if the funder provides guidance on developing a data sharing plan. The NIH provides guidance on the development of data sharing plans and has sample data sharing plans available for investigators applying for funding.
- Data Management Plans describe how the data will be collected, stored, protected, shared, and used during the course of the project.
- Data Sharing Plans describe how the data will be curated and made available to secondary users after data collection ends. Including data sharing plans in research project funding proposals helps researchers consider the time and cost of managing and sharing data and what is needed to facilitate data sharing. The content of data sharing plans may vary by research project and funding agency. At a minimum, a data-sharing plan should generally include a timeline for when data will be made available, the data and supporting documentation that will be provided, whether and how data will be de-identified, where data will be deposited, and whether access to data will be restricted.
References to data sharing may be applicable in other aspects of the funding application, such as:
- Human Subjects Protection. In the funding proposal, discuss the potential risks posed to participants by data sharing and how the rights and confidentiality of research participants will be maintained in light of potential risks. Make sure informed consent documents include a statement that addresses data sharing.
- Project Budget and Budget Justification. When writing the funding proposal, check whether the funding agency requires qualitative data sharing and whether the funder allows data sharing expenses to be included in the project budget. The NIH allows budgets to earmark funds to compensate for the cost of data sharing. When writing a data sharing budget, consider the cost of all data management activities, such as de-identifying data, executing a data sharing plan (if applicable), and depositing the data. Repositories can help with budget planning.
Project Timeline. Consider the funder’s expectations for when the final dataset, or subsets of the final dataset, must be made available to secondary users. Additionally, consider the data-sharing expectations of journals that may publish findings from the data. NIH policy expects that data will be shared no later than the time of an associated publication or the end of the project period, whichever comes first. Keep in mind that preparing qualitative research data for sharing can take considerable time and effort. Reserve appropriate time and resources for the data sharing process, and do not wait until the time of publication to begin preparing a data deposit. Prepare documents for the data deposit as they become available throughout the data collection process.
3. Find a Location for Shared Data
When planning a research project, identify where data will be shared. This step can be initiated before submitting a research proposal to a funder. Some funding agencies may require data to be shared in a specific repository or may provide recommendations for repositories where data can be shared. The NIH encourages using a data repository over institutional collections or journal servers. Common data-sharing locations include:
- Data Repositories are professional organizations that facilitate data archiving and sharing with secondary users. Funders and journals tend to prefer them. A professional repository is the best location for sensitive data. Repositories may be field-specific or contain subject-specific collections. Repositories typically curate data, ensure compliance with regulations and funding agencies, conduct disclosure risk assessment, and may provide guidance on de-identification. Datasets deposited in a repository are assigned a unique DOI and full citation when the data becomes available to secondary users. Researchers can cite datasets similarly to citing other references. Many repositories can track the impact of the dataset. There are a few repositories in the US that have experience curating and sharing English-language qualitative data:
- Inter-university Consortium for Political and Social Research (ICPSR)
- Qualitative Data Repository (QDR)
- Institutional Collections. Some institutions provide in-house archival services to researchers looking to deposit data. These collections are typically low-cost to the research team and assign a citation to the dataset. However, institutional collections vary from one institution to another in terms of options available for data curation, secondary user access restrictions, and impact tracking.
- Journals may allow or require data to be shared as supplemental files to a publication. Sharing data as supplemental files leaves the fewest options for researchers; data are not curated, are available open-access to secondary users without restriction, and the impact of the dataset is typically not tracked by the journal. Supplemental files are not an appropriate sharing option for sensitive data.
Key Considerations Appropriate locations for shared data will vary depending on the field of study, the sensitivity of the dataset, and the resources of the repository and study team. Here are a few key considerations for choosing an appropriate location for shared data:
- Funding Agency Requirements. Always check with the funding agency to determine their expectations for how data will be shared.
- Cost. Repositories may charge a fee for processing a data sharing agreement and curating deposited data, or they may charge fees for secondary users who access the shared data. Ask a repository curator for an estimate of the costs to deposit data and be aware of any additional fees that may be charged to secondary users.
- Support Services. Data repositories or institutional archives may be able to provide services or resources to the research team that makes the data-sharing process easier. This might include data curation or consultations with a curator who can provide expertise on the sensitivity of the dataset and advise on data de-identification. Journals do not typically provide these services.
- Secondary User Access. Secondary users must be able to discover the dataset and access it using a method that is appropriate for the sensitivity level of the data. Consider depositing data in subject- or field-specific collections to ensure that the data are likely to be found by relevant secondary users. Ask a repository curator whether data will be available open access or restricted access and what each would entail for secondary users.
4. Include Plans to Share in IRB Protocols
Include plans to share data in the IRB submission for a proposed study. The IRB may offer templates for data-sharing plans for investigators to include in their submissions. The informed consent documents of the study should include language informing participants of how the data will be used, stored, and shared with others in the long term, as well as how their confidentiality will be maintained. Avoid consent language that states the study data will be destroyed in the future, or that promises no one outside the research team will see the data. Check with IRBs, funders, and professional organizations for requirements to retain data for a certain number of years.
During her doctoral studies, Dr. Pope interviewed female sex workers in three southwestern states to understand their perceptions of health and body image. In her original consent form, she told participants that their data would not be shared with anyone outside the research team and all data would be destroyed after three years. Two years later, as a faculty member at the same institution, Dr. Pope secured funding to continue working with this population and would like to incorporate her previous data. However, her funder requires a data-sharing plan, and she is not sure she can share the original data without the participants’ consent.
Options for Resolution:
Ideally, Dr. Pope would have written data-sharing plans into her consent form. It is important to consider your data sharing plans as early as possible in your project design to avoid future sharing obstacles, and never promise to destroy data. However, at this point, two options exist: Dr. Pope can not share the data, or she can re-consent the original participants to allow for data sharing.
Dr. Henderson is a community-based participatory action researcher working closely with several participants to assess the effects of an after-school program on the violent crime rates among high school students in Chicago. Her participants include high school students, after-school program leaders, and law enforcement officials. When designing the study, Dr. Henderson’s contacts were enthusiastic about the project but wanted more formal involvement and recognition as study partners. Instead of using template consent form language that would require participants to be anonymous, they asked Dr. Henderson to revise the consent form and include their identities in all study findings in order to shed light on the important work happening in the community.
Options for Resolution:
Ideally, Dr. Pope would have written data-sharing plans into her consent form. It is important to consider your data sharing plans as early as possible in your project design to avoid future sharing obstacles, and never promise to destroy data. However, at this point, two options exist: Dr. Pope can not share the data, or she can re-consent the original participants to allow for data sharing.
5. Execute Data Sharing Agreements
If a data sharing agreement is required (e.g., data will be shared under restricted access), begin executing this agreement as soon as the study receives IRB approval.
A data sharing agreement is a legal agreement that determines how a repository will house and share deposited data with secondary users. A repository curator and institutional official can help determine whether a data sharing agreement is required. Execute data sharing agreements as early as possible in project planning. This process can take several months, depending on the home institution and the content of the data being deposited. Repositories often have template data sharing agreements that investigators can bring to their institutional officials. An institutional official is someone qualified to sign legal agreements on behalf of the institution. They are usually a member of the institution’s office for sponsored projects or research contracts office. Institutional Review Boards are generally only consulted when there is a question about whether participants consented to data sharing.
An institutional official can also help determine who owns the rights to a dataset. Generally, data collected at an institution are owned by that institution—not the investigator. Rules may differ for students who collect data as part of their degree programs. Data from news sources, legal documents, or other proprietary or publicly-available data may be owned by other parties. Institutional officials can help determine data ownership if there is uncertainty.
Dr. May recently joined a new institution and is getting ready to share data on pediatric patient experiences of living with a brain tumor. However, these data were collected at her previous institution, so she is unsure which institution owns the data and how to proceed with sharing.
Options for Resolution:
Dr. May should contact the institutional officials at her current and previous institutions to determine data ownership. They can determine who currently owns the data and how to proceed. A restricted-access data sharing agreement generally must be signed by an Authorized Official/Institutional Signatory
Dr. George is preparing to share her data on the experience of parents of children with autism. Her informed consent form did not address data sharing. Because she did not receive explicit participant consent to share, she wonders if she needs IRB approval to share data.
Options for Resolution:
In the future, Dr. George will want to address data sharing in the consent form. Dr. George should start by talking with her institution’s contract office (or the appropriate office to handle data-sharing agreements) to set up the appropriate legal agreements with her selected repository. Once the data is sufficiently de-identified, it may no longer be considered human subjects data and thus is no longer in the purview of the IRB. The contracts office could advise on whether the IRB needs to be consulted to share the data.
6. Account for Data Sharing in Project Management
Federal data sharing policies require data sharing at the time of first publication or at the end of the project period, whichever comes first. Sharing qualitative data can take significant time and effort, so don’t wait until the project’s end or the time of publication to start de-identifying data or preparing supplemental materials. Reserve time, effort, and budget for data-sharing activities, particularly for data de-identification. When developing data collection instruments, only collect data necessary to conduct the study. Avoid collecting identifiable information that will not be used for analysis.
Protocolize the Data Sharing Process. Incorporate data sharing plans in the data collection and management protocols that the study team will use to conduct the study, and keep this protocol updated throughout the project. Ensure that each member of the study team is aware of and trained to comply with the protocol. Here are some important elements to include in the study protocol:
- File Naming & Storage. Establish a standard file naming and storage system for the data and documentation required for deposit. File and folder naming conventions should be clear and indicate their contents, especially when differentiating between identifiable and de-identified versions of data files. Store data files in a format conducive to long-term preservation and, therefore, easily accessible by others for the foreseeable future. Consult with a repository curator on the best file format for study data.
- Data De-Identification. Set a data de-identification protocol that defines when and how data will be de-identified and how the de-identification process will be documented. This includes information about the types of Potentially Identifying Variables (PIVs) to be removed from the dataset, the text that PIVs are to be replaced with, the tools or services to be used for de-identification, and who is responsible for removing them. It is highly recommended to de-identify data during the data collection process as part of regular data cleaning activities. Waiting until the end of data collection or the complete end of a project to de-identify data can cause a project to run less efficiently, sometimes resulting in duplicated staff effort or delayed publication. Keep the data de-identification protocol updated throughout the data collection process, as it is likely to be adapted as new data is collected and reviewed by the study team. See our section on Data De-Identification for specific guidance and resources on de-identifying qualitative data.
- Supporting Documentation. Prepare supporting documentation for the data deposit as these materials become available. Set data variables that are succinct and well-defined in a codebook and store copies of consent forms and data collection instruments in project folders designated for the data deposit. Track any acronyms, abbreviations, or key terms in a data glossary to help secondary users understand the data.
7. De-Identify Data
The types of Potentially Identifying Variables (PIVs) that need to be removed from a dataset will depend on the sensitivity of the data and the context of the PIV in the transcript. If the repository offers curation services, a curator will likely review a deposit before making it available to secondary users to ensure the data are adequately de-identified. In community-based participatory research or similar community engaged research, working with communities to develop data de-identification strategies may be appropriate. It is also possible to offer participants the option of requesting that certain aspects of an interview be redacted after the interview is complete.
Determine What Information Is Identifiable in the Context of the Study.
It’s important to balance the need to protect participant privacy and confidentiality with the need to maintain the context needed for secondary users to understand the findings of the original study. Any information essential to data analysis must be kept in the dataset. Other information may not need to be removed simply because it is too general to be identifiable. This includes the names of fictional characters or public figures, nationwide or global organizations, or pseudonyms that have already been incorporated into the dataset. In rare cases, participants may consent to have their identifiable information shared when they participate in studies, in which case identifiable information about the participant does not need to be removed.
One good rule of thumb is to remove information that falls under HIPAA Safe Harbor. However, this may not be sufficient for all datasets, given that HIPAA Safe Harbor identifiers are not commonly present in qualitative data, and it may remove too much information from others. For example, in a study of employees evaluating their worksite health program, removing the employer’s name—which is not covered under the HIPAA Safe Harbor rule—may be required in addition to removing HIPAA Safe Harbors.
Dr. Nguyen is de-identifying his interview transcripts with nurses in Wyoming. He wonders how to strike a balance between de-identification and maintaining the integrity of the data. For example, only one of his participants is a male. The participant mentions being Vietnamese and working in a psychiatric unit. Dr. Nguyen’s de-identification protocol does not generally prohibit retaining the participant’s gender, nationality, or specialty in the transcript, but this combination of identifiers could make it easy to identify the participant.
Options for Resolution:
These details about the participant could be as identifiable as a name. Dr. Nguyen might consider which PIVs are the most identifiable and the least relevant to the analysis. In this case, the participant’s nursing specialty and nationality were irrelevant to the study purpose and analysis. Replacing the text with “Asian” and “nursing unit” might be a reasonable approach to blurring the original data. Keep in mind that de-identification is just one method of data protection. When data are sensitive or especially difficult to de-identify, the best practice is to use multiple methods of data protection, such as de-identification and restricted use agreements. The QuaDS Software is a helpful tool to use as a first step in flagging PIVs. In finalizing a dataset to share, researchers must consider the context and goals of their research and strike a balance between removing or replacing PIVs and preserving important contextual information. |
Comply with applicable rules, regulations, and agreements regarding the dataset. This may include HIPAA, the Common Rule, or agreements with community leaders.
Set standard rules for replacing PIVs in the dataset to ensure that replacement text is consistent and secondary users will understand the replacements. Always put replacement text within brackets to indicate where the transcript has been modified for de-identification. Use descriptive replacements that indicate what type of information was removed, and be consistent with text used to replace the same types of identifiable information, including exact synonyms. For instance, do not use [Midwestern University] and [Midwest Institution] interchangeably when replacing the name of a college, and similarly replace all name variations of the same entity, e.g., “Washington University” and “WashU” with the same text [Midwestern University].
When defining appropriate text replacements that are consistent and trackable, there are a few strategies that can balance the need for de-identification while ensuring that secondary users can sufficiently understand the context of the interview. Use the replacement strategy (or combination of strategies) most appropriate for the context of the transcript and the study:
- Contextual. Provide replacement text that describes the context of the interview. In the example below, the name Dr. Lee may be replaced with [Psychiatrist] as this preserves Dr. Lee’s relation to the participant. A contextual replacement strategy is appropriate for de-identifying most types of PIVs.
- Pseudonym. Create pseudonyms for certain types of PIVs, such as the names of people. In the example below, the names Emilio and Mateo have been replaced with [Juan] and [Carlos], respectively. Pseudonyms can be especially helpful for maintaining a sense of story by reducing the number of contextual replacements that can disrupt the flow of a transcript. Consider the pros and cons of adopting a pseudonym. Pseudonyms can preserve cues such as gender or cultural background that may be lost when a name is replaced with generic text such as [Name 1]. However, improperly using pseudonyms can risk stereotyping a participant or inaccurately changing their cultural identity.
- Broad Categorical. Define broad categories of replacement text that are replaced by a single standard replacement text, followed by a persistent number. The QuaDS Software uses a broad categorical strategy to replace text by default; however, it is recommended that users update these default replacements with contextual or pseudonym replacements. In the example below, Houston is replaced with [Location 1]. Note that later in the example, persistent numbering is used in combination with contextual replacements to distinguish between [Large Southern University 1] and [Large Southern University 2]. This indicates that the participant is talking about two different universities with similar contextual cues.
Original Text
“My twin brother Emilio has autism, but I don’t. Growing up in Houston, this meant that we qualified for a lot of twin studies at Texas Tech University. We were in this one autism study led by Dr. Lee that lasted most of our childhood. I’m a medical interpreter at TTUHSC now, and the other day I actually ran into Dr. Lee on campus. I stopped and told her that my brother and I had participated in her research, and as soon as I said that my name is Mateo she immediately remembered us and wanted to know how Emilio was doing. I couldn’t believe her memory was that good! She’s retired now, but apparently, she’s a consultant for some studies at Texas Tech and Baylor.”
Replacement Text
“My twin brother [Juan] has autism, but I don’t. Growing up in [Location 1], this meant that we qualified for a lot of twin studies at [Large Southern University 1]. We were in this one autism study led by Dr. [Psychiatrist] that lasted most of our childhood. I’m a medical interpreter at the [Large Southern University 1] medical center now, and the other day I actually ran into Dr. [Psychiatrist] on campus. I stopped and told her that my brother and I had participated in her research, and as soon as I said that my name is [Carlos] she immediately remembered us and wanted to know how [Juan] was doing. I couldn’t believe her memory was that good! She’s retired now, but apparently, she’s a consultant for some studies at [Large Southern University 1] and [Large Southern University 2]”
Establish a system for the study team to review and resolve questions that arise during the data de-identification process, and encourage team members to use this process any time they are unsure whether the text is identifiable or not. Reviewing questions involves having the study team member de-identifying the transcript discuss their question with another study team member, such as the team member who conducted the interview or the study’s principal investigator. Depending on the nature of the transcript and the dataset, resolutions to these questions may only apply to one transcript, or it may require updates to the data de-identification protocol and apply to all transcripts in the dataset.
EXAMPLE
Protecting participants from themselves…
Dr. Lane is preparing to share her data for her study on Covid-19 research practices. All participants agreed to data sharing and were willing to be identified. However, during data cleaning, Dr. Lane noticed one participant who complained about his sponsor and said harmful things about co-workers. She wonders whether she should redact this information. Although all participants agreed to data sharing in Dr. Lane’s study, it is still important to protect participants from potential harm that could result from sharing.
Options for Resolution:
In this case, Dr. Lane could remove certain sentences or paragraphs from the transcript that could have negative implications for the participant. Sometimes researchers may have to protect participants from themselves; if they say something harmful or potentially damaging, the researcher should remove this information, even if the participant agreed to data sharing and is named or could be identified.
Replace identifiable information consistently across linkable data sources. This may include survey data accompanying the transcripts, multiple transcripts collected from the same participant over time, or quotes provided in publications.
Linked data sources or individual transcripts may also include information that provides a cue to a PIV that was previously removed from the transcript. For instance, if a participant’s location in St. Louis was removed from linked survey data, but the participant later mentions “BreadCo,” in the transcript, this information needs to be removed. The reference to “BreadCo” is a term that individuals in St. Louis, Missouri, exclusively use to refer to the chain restaurant Panera.
If sharing qualitative and quantitative data from the same, mixed-methods study, consider whether linking the two data sets is necessary. For example, if the purpose of the quantitative data was to test certain assumptions or to characterize the overall sample, then it may not be necessary to link the datasets. However, in some cases—such as a sequential explanatory study—it may be necessary to link some quantitative and qualitative data, though perhaps only a subset—the subset of data that are explored sequentially.
- QuaDS Software. The QDS Project team developed the QuaDS Software as a tool to support qualitative data de-identification. The software reviews transcript data and flags potentially identifiable information, including all HIPAA safe harbor identifiers and a set of PIVs. PIVs include institution or organization names, race and ethnicity, LGBTQ identity or sexual orientation, all dates and ages, locations, and rare diseases or illnesses. It allows users to replace identifiable text throughout a transcript. The QuaDS Software identifies relevant variables with a precision score of .95 while maintaining a .96 recall score. While this performance is excellent, it still requires a human review to check the quality of de-identification and produce transcripts that are suitable for secondary use. A human can identify flagged words that are safe to leave in.
- Transcription Companies. Some transcription companies offer de-identification services as audio files are transcribed. What this means is not clear. Researchers will still need to review transcripts carefully.
- Data Repository Curator. A repository curator can provide consultation on whether information needs to be removed from a transcript and review a data deposit before it is made available to secondary users to ensure that the data is adequately de-identified.
8. Deposit Your Data
Once the de-identified data are available and all prior agreements have been approved, gather all de-identified data files and supporting documentation. Upload these materials to the data repository, and complete the deposit.
9. Track Data Sharing
Once the dataset is released for secondary use in a repository, it will be assigned a citation and persistent identifier, such as a DOI. The repository can also provide statistics on secondary user access to the data. Cite the dataset in relevant publications and professional bibliographies, and track the metrics on secondary user statistics.
ADDITIONAL RESOURCES
These repositories in the US have experience curating and sharing English-language qualitative data:
- Inter-university Consortium for Political and Social Research (ICPSR)
- Qualitative Data Repository (QDR)
For researchers working in contexts outside of the US, these repositories have extensive experience curating qualitative data:
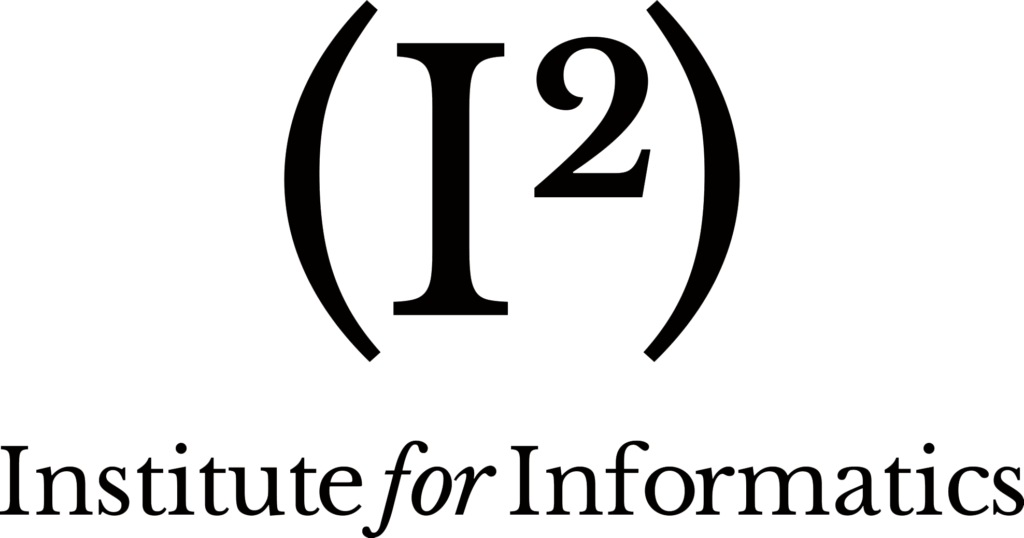
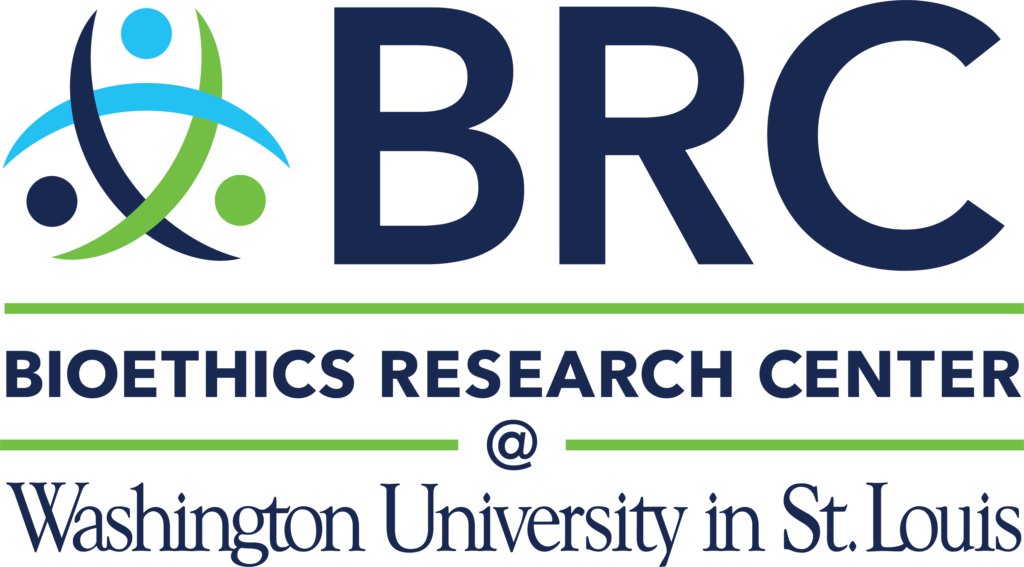
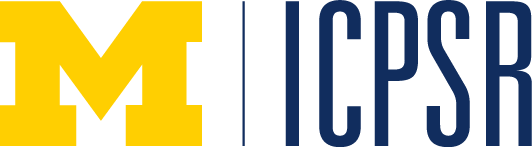
© Copyright Bioethics Research Center 2023